Research Topic 2
Research Topic 2 (RT2) investigates the field of Computational Fluid Dynamics (CFD), which studies and simulates how fluids like air and water move and interact with other objects or with each other, through numerical computation algorithms. CFD is essential for modeling industrial, physiological, and environmental processes, thus enabling the realization of their Digital Twins. Yet, the subtleties of fluid dynamics pose considerable computational hurdles.
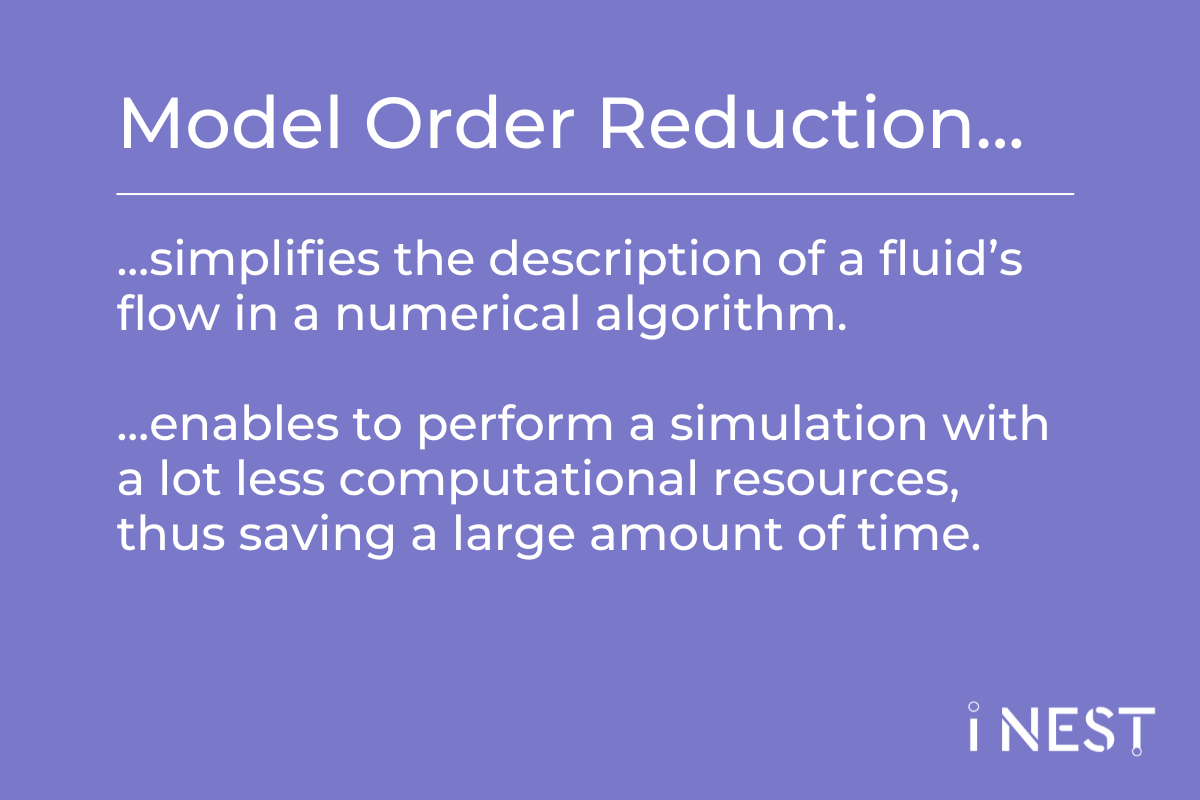
RT2 approaches this challenge by exploring Model Order Reduction (MOR) techniques. By keeping only the most relevant parameters in a model, MOR techniques offer a streamlined yet accurate portrayal of fluid behavior. MOR thus enables to speed up algorithm execution, paving the way for real-time simulation.
However, every simplification introduces new approximations and, consequently, potential errors—this is also the case with MOR techniques. That is why Uncertainty Quantification becomes even more crucial: only through this can the Digital Twin remain a faithful and reliable representation of the process or product it aims to replicate.
MOR techniques: some remarkable achievements

Naval Industry
Reduction of fuel consumption and pollution in passenger ships.
Optimization of ship structures.
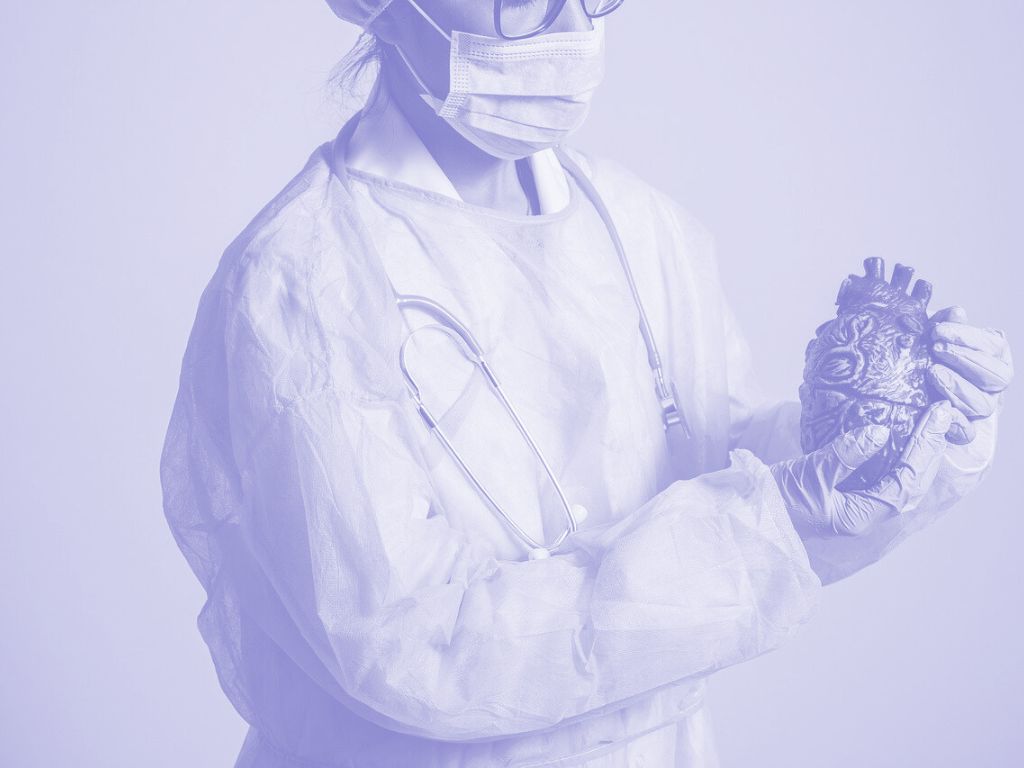
Medicine
Real-time patient-specific diagnostics for cardiovascular diseases.
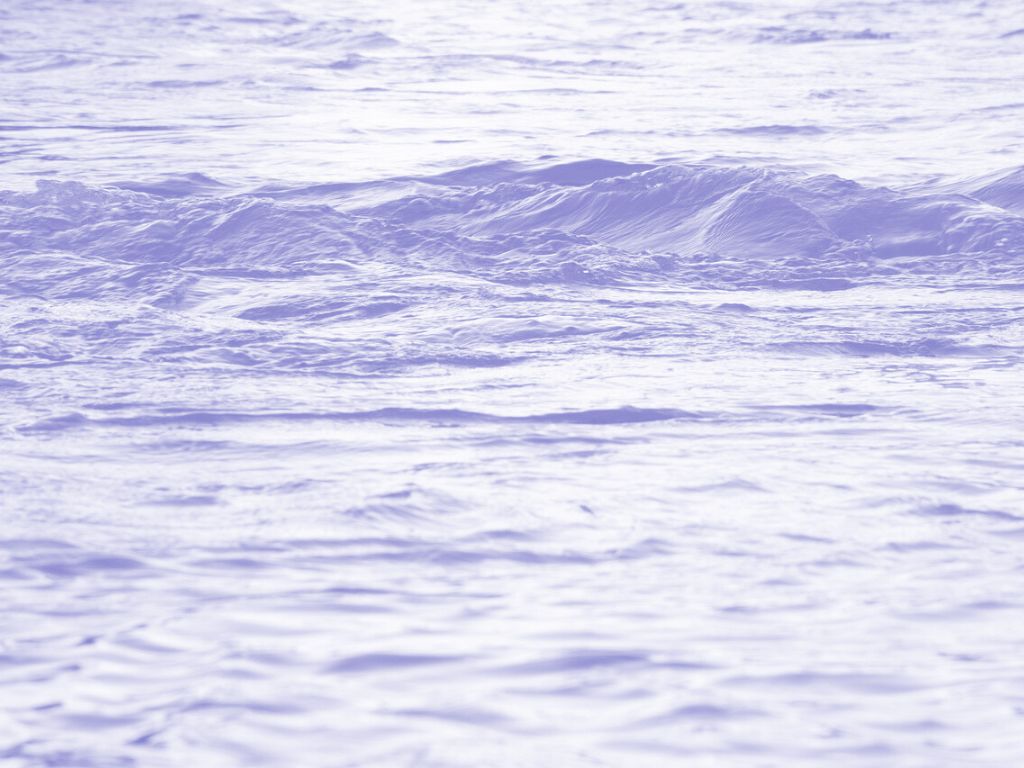
Environment
Study of the impact of pollutant diffusion in the Gulf of Trieste on marine ecosystems.
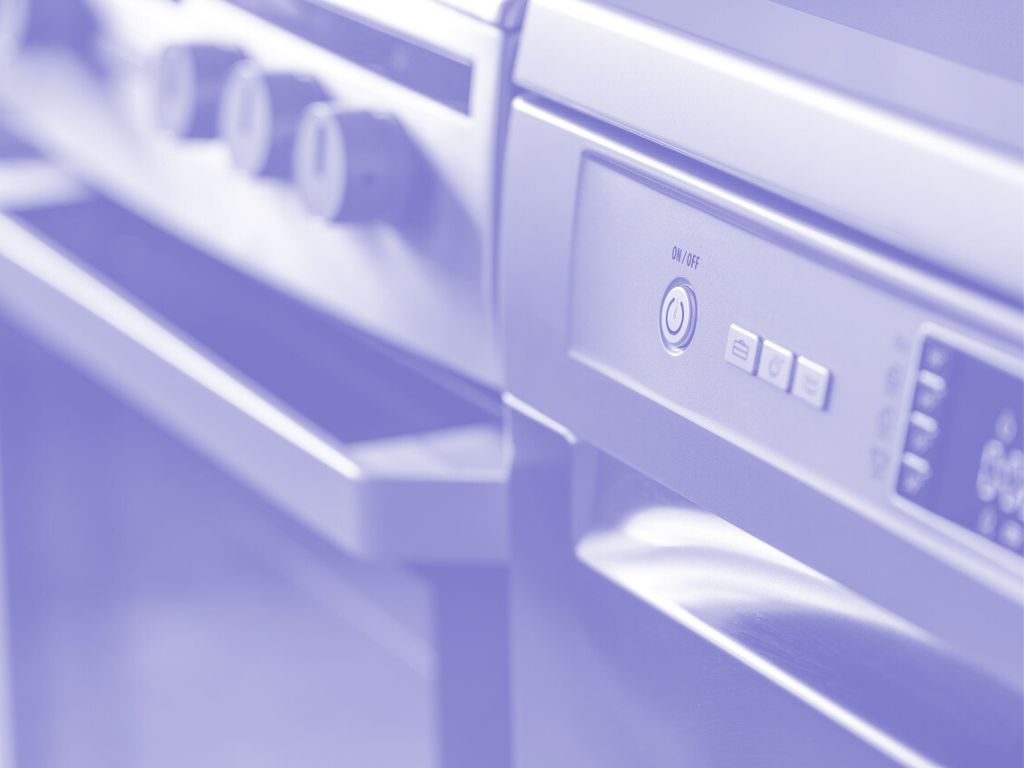
Other sectors
Appliance optimization.
Food waste minimization.
Early detection of structural damages in tunnels.
Coordination
Research Topic 2 is led by the International School for Advanced Studies (SISSA). The University of Padua (UniPD) and the National Institute of Oceanography and Experimental Geophysics (OGS) are also involved in RT2.